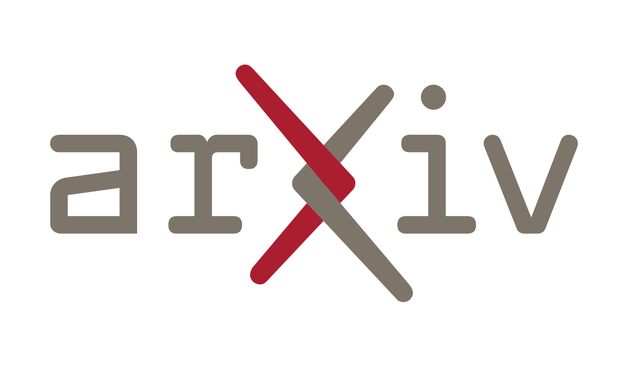
arXiv.orgPsOCR: Benchmarking Large Multimodal Models for Optical Character Recognition in Low-resource Pashto LanguageThis paper evaluates the performance of Large Multimodal Models (LMMs) on Optical Character Recognition (OCR) in the low-resource Pashto language. Natural Language Processing (NLP) in Pashto faces several challenges due to the cursive nature of its script and a scarcity of structured datasets. To address this, we developed a synthetic Pashto OCR dataset, PsOCR, consisting of one million images annotated with bounding boxes at word, line, and document levels, suitable for training and evaluating models based on different architectures, including Convolutional Neural Networks (CNNs) and Transformers. PsOCR covers variations across 1,000 unique font families, colors, image sizes, and layouts. A benchmark subset of 10K images was selected to evaluate the performance of several LMMs, including seven open-source models: DeepSeek's Janus, InternVL, MiniCPM, Florence, and Qwen (3B and 7B), and four closed-source models: GPT-4o, Gemini, Claude, and Grok. Experimental results demonstrate that Gemini achieves the best performance among all models, whereas among open-source models, Qwen-7B stands out. This work provides an insightful assessment of the capabilities and limitations of current LMMs for OCR tasks in Pashto and establishes a foundation for further research not only in Pashto OCR but also for other similar scripts such as Arabic, Persian, and Urdu. PsOCR is available at https://github.com/zirak-ai/PashtoOCR.