https://medium.com/@Joekbennett/diffusing-ghibli-0f5a6987fcf2?source=rss------machine_learning-5
#diffusion-models #ai #machine-learning
Event Attributes
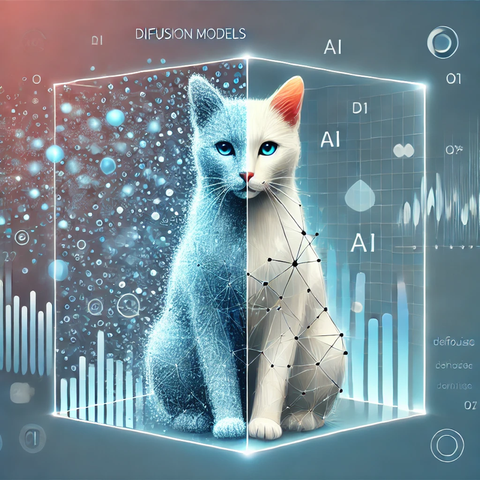
Revolutionizing 3D Scene Generation: Meet Bolt3D
In a groundbreaking leap for 3D modeling, Bolt3D achieves rapid scene generation in just 6.25 seconds using a single GPU. By leveraging advanced diffusion models and Gaussian regression techniques, th...
https://news.lavx.hu/article/revolutionizing-3d-scene-generation-meet-bolt3d
If, like me a few months ago, you have installed ComfyUI and are working out what next, then this guide walks you through a basic workflow and helps put some sense to all the settings and basic nodes. #AI #ComfyUI #Flux #DiffusionModels #ImageGeneration
Si può ottenere un programma funzionante partendo da un testo casuale? Pare di sì, o almeno questo sostengono gli autori di Mercury-Coder, un sistema per creare programmi che non opera come i normali LLM. Sarà davvero più veloce a parità di precisione? Provare per credere! #ai #intelligenzaartificiale #programming #diffusionmodels #mercurycoder https://www.youtube.com/watch?v=rU-PfRJN0xA
Microsoft’s New BioEmu-1 AI Model Can Predict How Proteins Move and Change
#AI #BioEmu1 #Microsoft #MicrosoftResearch #DiffusionModels #BioScience #Science #AIResearch #Alphafold #BigTech
Revolutionizing Language Generation: The Rise of Diffusion Large Language Models
Inception Labs has unveiled a groundbreaking approach to language generation with its diffusion-based models, promising unprecedented speed, efficiency, and quality. This innovative technology not onl...
Inception Unveils Revolutionary Diffusion-Based Language Model for AI
Inception, a startup founded by Stanford's Stefano Ermon, is revolutionizing the AI landscape with its diffusion-based language model (DLM). Promising tenfold improvements in speed and cost efficiency...
https://news.lavx.hu/article/inception-unveils-revolutionary-diffusion-based-language-model-for-ai
Breaking the Curse of Dimensionality: Insights from Diffusion Models in Machine Learning
Recent research demonstrates how diffusion models can effectively learn low-dimensional distributions, revolutionizing the way we approach image data in machine learning. This article delves into the ...
Revolutionizing Language Models: The Rise of Large Language Diffusion Models
In a groundbreaking study, researchers introduce LLaDA, a diffusion model that challenges the dominance of autoregressive models in large language processing. With impressive benchmarks and novel meth...
Unleashing the Power of AI: Building a Real-Time Snake Game with Diffusion Models
Imagine playing a classic Snake game, but this time, it's powered by cutting-edge AI diffusion models. The Snake Diffusion project not only revives nostalgia but also showcases the potential of machin...
Revolutionizing Video Generation: Meet Diffusion as Shader (DaS)
In a groundbreaking development, researchers unveil Diffusion as Shader (DaS), a game-changing approach that empowers users to control video generation like never before. By harnessing 3D tracking, Da...
https://news.lavx.hu/article/revolutionizing-video-generation-meet-diffusion-as-shader-das
Revolutionizing Video Generation: Meet Diffusion as Shader (DaS)
In a groundbreaking development, researchers unveil Diffusion as Shader (DaS), a game-changing approach that empowers users to control video generation like never before. By harnessing 3D tracking, Da...
https://news.lavx.hu/article/revolutionizing-video-generation-meet-diffusion-as-shader-das
New paper: ‘An Analytic Theory of Creativity in Convolutional Diffusion Models’ explores how diffusion models generate creative outputs by combining training data patches. A groundbreaking foundation for understanding generative AI.
#AI #GenerativeAI #DiffusionModels
https://arxiv.org/abs/2412.20292
[synthetic media] OpenAI: Sora text-to-video
Sora: https://sora.com
Sora is here | OpenAI: https://openai.com/index/sora-is-here/
https://news.ycombinator.com/item?id=42368604
Sora (text-to-video model)
https://en.wikipedia.org/wiki/Sora_(text-to-video_model)
Diffusion Models are Evolutionary Algorithms
https://arxiv.org/abs/2410.02543
https://old.reddit.com/r/MachineLearning/comments/1fzbvq3/r_diffusion_models_are_evolutionary_algorithms
* diffusion models inherently perform evolutionary algorithms
* naturally encompass selection, mutation, reproductive isolation
Diffusion model: https://en.wikipedia.org/wiki/Diffusion_model
Stable Diffusion: https://en.wikipedia.org/wiki/Stable_Diffusion
Evolutionary algorithm: https://en.wikipedia.org/wiki/Evolutionary_algorithm
Genetic programming: https://en.wikipedia.org/wiki/Genetic_programming
I wrote a thread last week on LinkedIn in which I discussed these three articles and explained how they fit together in a broader context. Rather than repeating the thread here, I will just link to it:
https://www.linkedin.com/posts/lthornemccarty_machinelearning-deeplearning-generativeai-activity-7197269535287058434-4d6l?utm_source=share&utm_medium=member_desktop
Comments and questions are welcome!
Scientists have devised a technique called "#DistributionMatchingDistillation" (DMD) that teaches new #AIModels to mimic established image generators, known as #DiffusionModels, such as #DALLE3, #Midjourney and #StableDiffusion.
This framework results in smaller and leaner #AI models that can generate images much more quickly while retaining generated image quality.
MIT scientists have just figured out how to make the most popular #AIImageGenerators 30 times faster
https://www.livescience.com/technology/artificial-intelligence/mit-has-just-worked-out-how-to-make-the-most-popular-ai-image-generators-dall-e-3-stable-diffusion-30-times-faster